Table Of Content
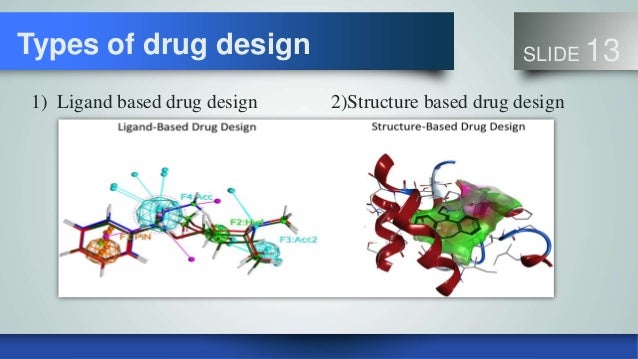
This includes, but is not limited to, Aliskiren, Boceprevir, Captopril, Dorzolamide, Nolatrexed, Oseltamivir, Rupintrivir, Saquinavir and Zanamivir [2]. 5In the ligand optimization stage of CADD, as only a few compounds are under consideration, accuracy rather than computational efficiency is usually pursued. It uses all-atom explicit-solvent MD simulations that include small organic solutes, such as propane, methanol and others, to identify 3D functional-group binding patterns on the target.
Computational approaches to drug design
While FEP and molecular mechanics (MM) with Poisson–Boltzmann (PB) and surface area solvation (MM/PBSA) as well as MM/generalized-Born SA (GBSA) methods (107) do account desolvation, these are computational demanding approaches that limits their utility in CADD. A novel method designed to overcome this drawback is the SILCS-MC (19) docking method put forward by our laboratory. This takes advantage of the use of GCMC/MD simulations of the protein in aqueous solution with selected organic solutes to precompute the GFE FragMaps that are free energy functional group affinity patterns that encompass the entire protein and account for protein flexibility and desolvation contributions (108–110).
Challenges of gigascale screening
This technique uses a known active compound as a query compound to find similar compounds and then rank compounds identified in a database. Based on this belief, structurally similar molecules exhibit similar biological activities and physicochemical properties. Numerical descriptors are applied and similarity coefficient is defined to quantify the degree of similarity (similarity/ dissimilarity). A number of coefficients are applied in similarity searching with different fingerprint molecular databases (Cosine, Euclidian distance, Forbes, Tanimoto coefficients etc.). 3Protonation states of titratable residues at the targeted binding site and in the ligand being studied are quite important when setting up the CADD calculations.
AI in Drug Discovery: Trust, but Verify - Genetic Engineering & Biotechnology News
AI in Drug Discovery: Trust, but Verify.
Posted: Thu, 11 Jan 2024 08:00:00 GMT [source]
Fundamental steps used in pharmacophore modeling

In the 1990’s, a large number of developments were undertaken using combinatorial and high-throughput screening technologies, which accelerated drug discovery [13-15]. These technologies were widely adopted because they enabled the rapid synthesis and screening of large libraries, but unfortunately, no significant success was achieved and little progress toward the development of new molecular entities was made [16, 17]. Dr. Scott R. Lokey is a Professor of Chemistry in the Department of Chemistry and Biochemistry at the University of California, Santa Cruz. His research group focuses on the relationship between molecular structure and drug-like properties, especially cell permeability. Professor Lokey is also the director of the UCSC Chemical Screening Center, a high-throughput screening facility dedicated to early stage lead discovery, and is co-founder of Circle Pharma, a biotech startup focusing on the discovery of cyclic peptide inhibitors against intracellular targets. Any lead compound can be modified structurally and maintain its physiochemical properties, thermodynamic, pharmacokinetic parameters, and toxicity (Jogensen, 2009).
Aurigene Pharmaceutical Services Ltd. introduces Aurigene.AI™, an artificial intelligence (AI) and machine learning ... - Yahoo Finance
Aurigene Pharmaceutical Services Ltd. introduces Aurigene.AI™, an artificial intelligence (AI) and machine learning ....
Posted: Wed, 03 Apr 2024 07:00:00 GMT [source]
Artificial Intelligence For Drug Development and Discovery Market 2022
The existing modern drug discovery implies the knowledge of genomics, bioinformatics, computational chemistry, and combinatorial chemistry for virtual screening, HTS, Lenovo ligands. However, the concept of LBDD relies mostly on drug planning, which develops a simple drug molecule, a 3D through trial and error methods that improves the overall biological activity of the compound. Although bioinformatics and molecular dynamics approaches can help to detect and analyse allosteric and cryptic pockets133, computational tools alone are often insufficient to support ligand discovery for such challenging sites. The cryptic and shallow pockets, however, have been rather successfully handled by fragment-based drug discovery approaches, which start with experimental screening for the binding of small fragments.
Ligand-Based Drug Design
In collaborative studies with the Wilks lab, we have successfully applied CADD techniques to identify inhibitors of the bacterial heme oxygenases from Pseudomonas aeruginosa and Neisseria meningitides, thereby confirming the potential role of heme oxygenases as a novel antimicrobial targets (13, 14). Computational binding site identification methods can be used to exploit novel, druggable sites on new protein targets for potential therapeutic development (113). For antibiotics development, such methods can be employed to search for putative allosteric sites as alternatives to the active or orthosteric sites on bacterial proteins to overcome drug resistance issues (114).
Other parameters like kinetics include thermodynamics, pharmacodynamics along with higher improvement in ADMET properties have also to be considered. Unfortunately, CADD suffers from cognitive dissonance (bias to seek consonance) and also sloppy research that results from the lack of proper training. The utility of the SSFEP approach is that the ΔΔG values for many modifications may be rapidly evaluated as the same trajectories from the original MD simulations of the hit compound are used in each case. This approach may be of use during the fine tuning of ligand affinity or specificity for a target or as required to improve physiochemical and pharmacokinetic properties without significantly altering desirable properties such as affinity. Docking involves posing a compound in the putative binding site on the target in an optimal way defined by a scoring function in combination with a conformational sampling method (78). Various docking programs are available that differ based on the scoring function used to describe the interaction between small molecule and the target and the conformational sampling method used to generate the binding poses of the ligand on the protein.
1. Structure-based Drug Design (SBDD)
Computer-aided drug design has helped to expedite the drug discovery and development process by minimizing the cost and time. In this review article, we highlight two important categories of computer-aided drug design (CADD), viz., the ligand-based as well as structured-based drug discovery. Various molecular modeling techniques involved in structure-based drug design are molecular docking and molecular dynamic simulation, whereas ligand-based drug design includes pharmacophore modeling, quantitative structure-activity relationship (QSARs), and artificial intelligence (AI).
CADD can be separated into ligand or hit identification and ligand or hit optimization, with both SBDD and LBDD methods useful in the appropriate context. Database screening methods are often used for hit identification (59) while a number of methods may be used for hit optimization (4, 24, 60). Below we present a collection of methods that may be used for both ligand identification and optimization. Computers have become an important part of every lab and drug discovery or designing processes are no exceptions. Based on that, it designs molecules that bind specifically to the protein according to the lock-and-key principle so they can interact with it.
Different methods employed in the identification of new inhibitors from chemical databases include pharmacophore modeling, quantitative structure-activity relationship (QSAR), molecular docking, quantum mechanics, and statistical learning methods. CADD can be broadly divided into structure-based and ligand-based drug design approaches, both have been widely used in the drug discovery process in the identification of suitable lead molecules. While the structure-based drug design relies on the three-dimensional structure of the target receptor and its active sites to understand the molecular interaction between the receptor and ligand, the ligand based-drug design depends on the knowledge of ligands interacting with the given target receptor [9]. Computer-aided drug design has a large number of success stories and continues to play a vital role in the drug discovery process [10]. In this regard, the approach has been utilized in proposing drug candidates against coronavirus disease 2019 (COVID-19).
The successful applications, limitations and future prospects of this approach have also been discussed. Pharma companies amass collections of compounds for screening in-house, whereas in-stock collections from vendors (see the figure, part a) allow fast (less than 1 week) delivery, contain unique and advanced chemical scaffolds, are easily searchable and are HTS compatible. However, the high cost of handling physical libraries, their slow linear growth, limited size and novelty constrain their applications.
Conversely, interactions of electrons with the nuclei of the compounds should be considered in small systems which require the application of quantum mechanics calculations. Quantum mechanics are based on quantum physics and very accurate electronic properties can be predicted using the appropriate level of theory and basis set. For instance, electron potential maps and frontier molecular orbitals and several parameters related with to them (chemical hardness, softness, ionization potential etc.) can be calculated.
In this process the available information of ligands and their biological activity is used for the development of new potential drug candidates. LBDD is widely used in pharmaceutical research, as more than 50% of approved drugs targeting membrane proteins (for which 3D structures are often not available, such as, GPCR). It is based on the assumption that compounds with similar structural features share common biological activities and interact/inhibit common target molecules [36, 37]. Running such data-rich computationally driven pipelines requires overarching data management tools for drug discovery, many of them being implemented in pharma and academic DDD centres146,147. Building computationally driven pipelines will also help to reveal weak or missing links, in which new approaches and additional data may be needed to generate improved models, thus helping to fill the remaining computational gaps in the DDD pipeline.
Some of the hardest cases are targets with cryptic or shallow pockets that have to open or undergo a substantial induced fit to engage ligand, as often found when targeting allosteric sites, for example, in kinases or GPCRs, or protein–protein interactions in signalling pathways. To answer those questions, we focus on structure-function, dynamics, and pharmacology of the GPCR superfamily, probing it with state-of-the-art structural bioinformatics and molecular modeling tools, and validating the predictions with experimental data from public sources and collaborators. Before we come to our topic of computer-aided drug designing, let's first introduce what is drug discovery. Over the years it has become clear that despite advances made in experimental mutational studies, a quantitative understanding of enzyme catalysis will not be possible without the use of computer modeling approaches. While we believe that electrostatic preorganization is by far the most important catalytic factor, convincing the wider scientific community of this may require the demonstration of effective rational enzyme design. Here we make the point that the main current advances in enzyme design are basically advances in directed evolution and that computer aided enzyme design must involve approaches that can reproduce catalysis in well-defined test cases.
No comments:
Post a Comment